Research
Core Aspects

Embedded Machine Learning
Artificial Intelligence has the potential to process high-dimensional data very efficiently. We investigate how concepts of Deep Neural Networks or Convolusional Neural Netowrks can be applied to embedded systems.
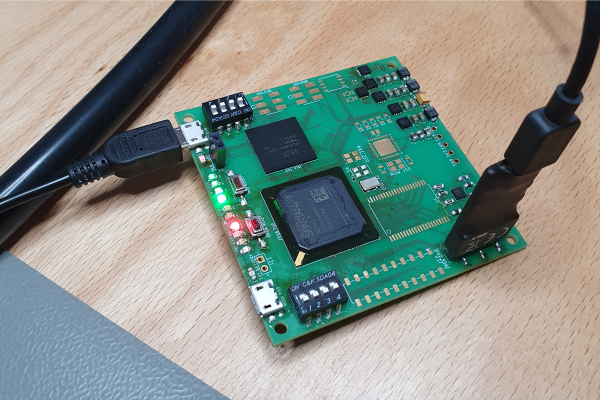
Programming Adaptive Systems
To cope with the rising performance requirements of future embedded systems we are developing new energy efficient hardware- and software solutions. We specifically focus on devices that incorporate reconfigurable and adaptive hardware.

IoT Deployment and the Edge
IoT systems often consist of a great number of networked embedded devices combined with a number of software services in the Cloud and Edge. Management and control of such highly distributed systems is a interesting challenge for IoT systems.
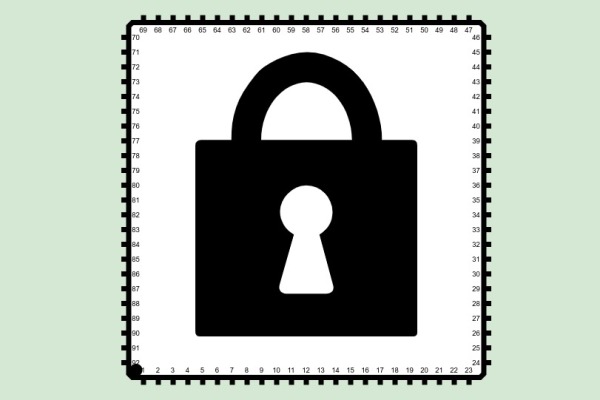
Embedded Security
IT security is a special challenge for embedded system, due to their limited amount of resources. A potential solution for IoT applications is Physically Unclonable Functions (PUF) on FPGAs.

Network Function Virtualization
Future network architectures are expected to meet diverse service requirements. One way to overcome these challenges is to adopt Network Function Virtualizatio, Software-Defined Networking and Multi-access Edge Computing.
Funded Projects
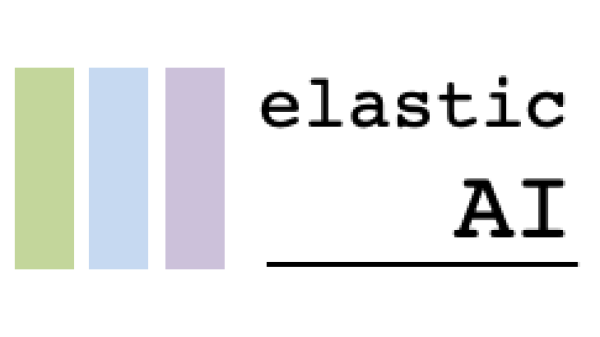
elastic AI
The elastic AI ecosystem creates development tools and runtime environments for both embedded and distributed AI applications.

Neural signal processing using artificial intelligence on an embedded platform (Sp:AI:ke)
How can AI be used in medical implants? The MERCUR project "Neural signal processing using artificial intelligence on an embedded platform" or Sp:AI:ke for short is tackling this question.

Completed Projects
- DAAD-funded Summer School in Armenia (2022)
- KI-LiveS (2019-2022)
- LUTNet (2019-2020)
- FiPS (2013-2016)
- Third Life Project (2015)